More Than a Sudden Leap in AI Image Processing
On March 29, 2025, In late March 2025, something extraordinary happened in the world of artificial intelligence. Within hours, two major players—OpenAI and Google—unveiled new ways to handle images using their AI systems. These announcements weren’t just about making pretty pictures; they marked a shift in how AI can work with visuals, moving beyond the methods that dominated the past few years. Until recently, creating or editing images with AI often meant using separate tools, like those behind the stunning artworks flooding social media. Now, that’s changing fast.
With the new methods, the same large language models (LLMs) or the powerful AI systems behind sophisticated chatbots are tweaked to generate and process images. It signaled a move towards a unified AI experience where understanding and manipulating images becomes as natural and integrated as writing an email or asking a question to a search engine. This seemingly subtle change represents a significant leap, suggesting that the era of specialized AI models for different tasks might be drawing to a close, replaced by a more versatile and all-encompassing architecture: the transformer.
Beyond the Hype: Image Generation as a Core AI Function
Photoshop has ruled the image-editing world for over 30 years, a tool so iconic it’s practically a verb. But AI is knocking on its door, and the gap between them is shrinking fast. In 2022, AI systems could barely muster basic edits—think blurry fixes or awkward color tweaks. The following table provides a glimpse of the pace of improvement through a subjective rating on a handful of features. Our AI models rank OpenAI’s latest 6.5 to 8.5 on overall abilities compared to Photoshop now. This is perhaps generous, but whether that’s true or a bit of hype, one thing’s certain: AI is getting better at an astonishing rate.

At the moment, chat-based AI systems can’t match Photoshop in areas like live editing or handling multiple images at once, known as batch processing. However, a major shift is underway. The diffusion method—a standalone technology that’s dazzled us with stunning image generation over the past two years—is being overtaken by transformers, the powerhouse behind these new AI advances. This change promises rapid improvements across the board. Tools built on older methods, including not just MidJourney but even Photoshop, face a double challenge: they’re at risk of losing their edge in the areas where they shine, while struggling to keep up with the fresh capabilities—like seamless integration and natural language control—that transformer-based systems bring to the table.
This rapid rise doesn’t mean Photoshop’s days are numbered. It’s still the choice for pros who need total control. But AI’s simplicity and speed are shaking things up for many of its business segments, offering a new way to work with images that fits our fast-paced lives.
The Rise of the Transformer: One Architecture to Rule Them All
Behind this image-editing boom lies a bigger story: Transformers are taking over AI, pushing aside older methods across all machine learning domains.
Here’s how the focus of AI research has shifted, based on recent studies:
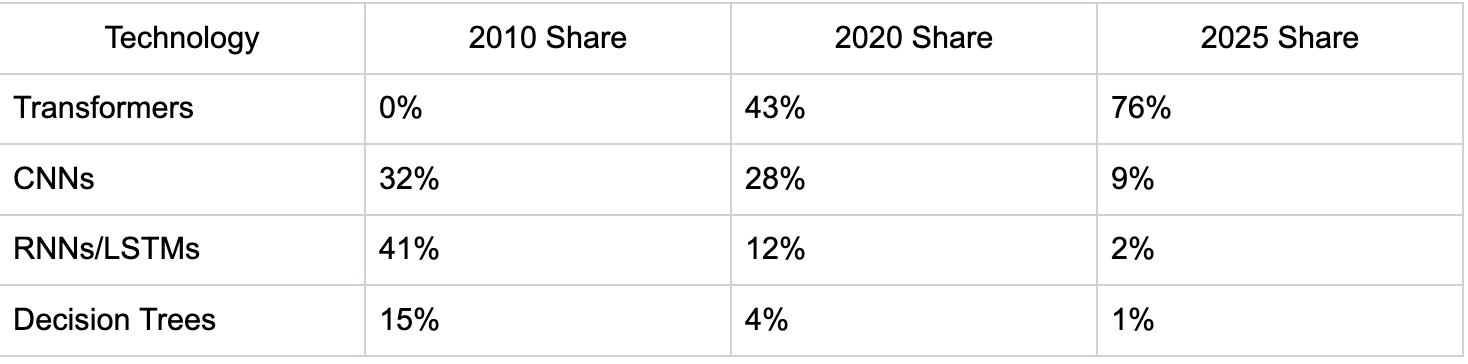
In actual life, the present status looks more like below:
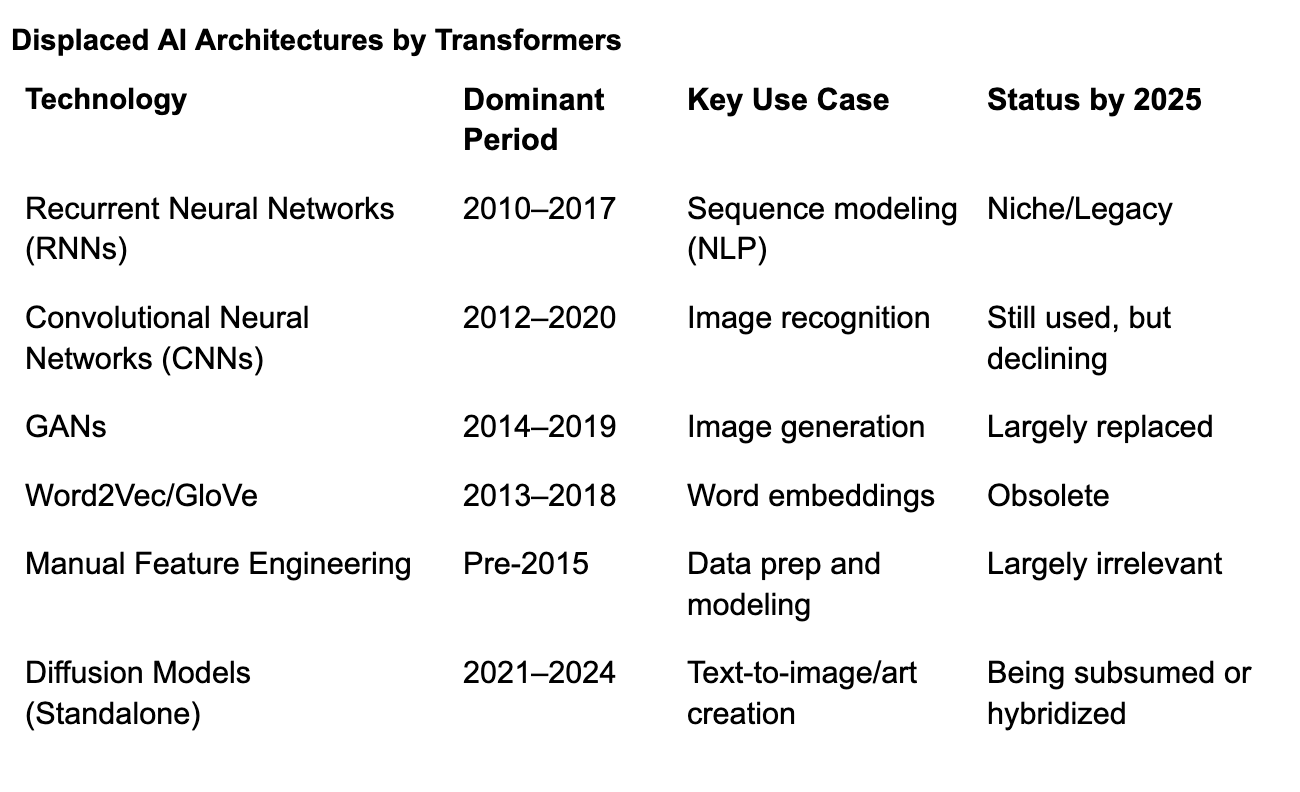
Shifting gears isn’t simple for companies or individuals who’ve spent years crafting their businesses around technologies from the last decade. Many of these players attempt to layer transformer-based applications onto their existing setups. This approach isn’t just awkward; it completely backfires. To understand why transformers require the destruction of the existing setup, let’s look at their issues with structured data.
The Struggle of the Structured World
Imagine one is running Salesforce with its massively successful product for decades. Along comes a new technology like transformers. The obvious business reaction is to layer on an AI feature, like what the company did with Einstein AI, to enhance what’s already there. The same strategy is deployed by Microsoft weaving Copilot into Office tools, SAP creating Joule, or Adobe embedding Firefly. Yet, these attempts have fallen flat. To understand the struggles of all sorts of companies in “adding AI,” we must understand how transformers require their data to realize the full potential.
For decades, organizing information into neat tables and databases was the key to making sense of it. Companies like SAP and Oracle built empires on this idea, sorting everything into rows and columns. But transformers thrive on the opposite: raw, messy, unstructured data—think emails, photos, or doctor’s notes scribbled in haste.
This shift is massive. Back in the 1980s, nearly all data processing relied on structured formats—think 10% unstructured at best. By 2025, that’s flipped to 60%, and it’s climbing. Transformers can dig into this chaos—text, images, videos—and pull out insights without needing everything pre-sorted. There is an increasing pile of examples that when forced to work with human classifications, categories or structures in any data, transformers’ usefulness declines.
Take healthcare: old systems used coded forms to track illnesses, but they missed rare conditions buried in freeform notes. A 2024 study found that transformers analyzing those notes boosted early detection by 41%. Diagnostics is undergoing a silent revolution as machines go past the human classifications to see patterns our previous methods could not. In multiple cancer detections, the false identifications have begun to plunge. Whether in sentiment scores or credit scores, transformers are proving more effective when provided all sorts of data without any structure.
Stuck in the Middle: The Incumbent Dilemma
Established players are in a bind. They can’t fully embrace transformers without cannibalizing the very businesses that made them dominant. For decades, their value lay in structuring data, designing control panels with sliders, toggles, and form fields—tools that gave the illusion of precision through rigid constraint. But transformers don’t need those structures. They operate better in the wild.
So while Adobe or SAP can add AI features, their core logic remains unchanged. The transformer revolution isn’t additive—it’s substitutive. It replaces how software works, not just what it does. And that’s precisely why these incumbents are vulnerable: too invested in the old, too cautious to bet on the new. The result? They’re stuck—unable to tear down what still brings in revenue, even as the future moves on without them.
One model is eating the world—and it’s not asking permission.